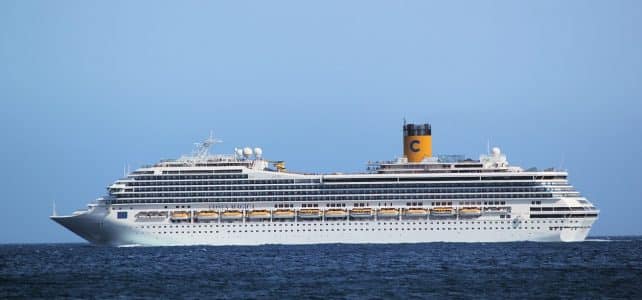
Costa Croisières est l’une des compagnies de croisières les plus populaires au monde, offrant des voyages extraordinaires à travers les mers et les océans. Mais quel est le plus petit bateau de la flotte de Costa croisière ? Découvrons ensemble…
Blog
Costa Croisières est l’une des compagnies de croisières les plus populaires au monde, offrant des voyages extraordinaires à travers les mers et les océans. Mais quel est le plus petit bateau de la flotte de Costa croisière ? Découvrons ensemble…
La Peugeot 2008, crossover urbain populaire, séduit par son design et ses performances. Comme tout véhicule, elle n’est pas à l’abri de problèmes occasionnels, allant de soucis d’éclairage à des dysfonctionnements moteur plus sérieux. Les propriétaires peuvent être confrontés à…
L’Europe, avec son riche héritage culturel et architectural, abrite aussi des temples modernes de la consommation qui méritent le détour. Au-delà de simples lieux d’achat, certains centres commerciaux se distinguent par leur conception innovante, leur offre pléthorique et leurs services…
Les nains, avec leurs corps compacts et leurs traits distinctifs, captivent l’imagination collective depuis des siècles. Ces êtres, souvent évoqués dans les récits fantastiques et les légendes anciennes, possèdent une biologie et une sociologie qui suscitent la curiosité scientifique. Leur…
Face à la digitalisation croissante des supports éducatifs, les collégiens de Seine-et-Marne se heurtent parfois à des obstacles limitant leur accès aux ressources pédagogiques en ligne. Que ce soit en raison de disparités matérielles, de lacunes dans la maîtrise des…
L’éventail des techniques diagnostiques en médecine est vaste et en constante évolution, chacune offrant des avantages spécifiques pour déceler des conditions médicales variées. Au cœur de ces méthodes, l’aspiration par aiguille fine (FNA) se distingue comme une procédure minimale invasive,…
L’Hallux Rigidus, une forme d’arthrite touchant l’articulation de base du gros orteil, peut être une source de douleur et d’inconfort au quotidien. Pour ceux qui en souffrent, le choix des chaussures n’est pas à prendre à la légère. Des critères…
La belote, ce jeu de cartes emblématique, rassemble les amateurs de stratégie et de convivialité autour d’une table. Or, pour les néophytes, l’aspect le plus intimidant peut souvent être le calcul des scores, une composante fondamentale pour mener son équipe…
Vous avez décidé de partir en croisière avec Costa Croisières et vous souhaitez réserver des excursions pour découvrir les destinations que vous allez visiter ? Pas de panique, nous vous expliquons tout ce que vous devez savoir pour réserver facilement…